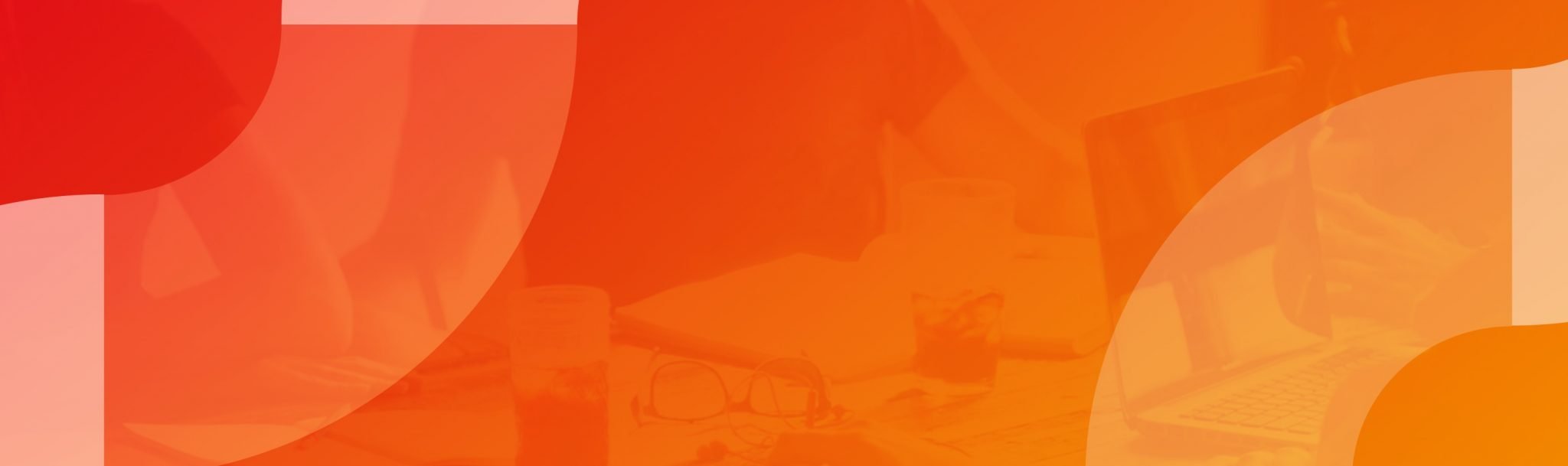
Where is the sweet spot for edge computing?
Any situation that requires a blend of local compute-like and cloud compute-like capabilities would benefit the most by using edge computing. Such use cases include, but are not limited to, advanced predictive maintenance, remote patient monitoring and video analytics for security.
The edge computing sweet spot: what makes an edge use case?
The main objective of edge computing is bringing cloud capabilities closer to the data source. This enables edge computing to provide cloud capabilities while also retaining local compute performance. Some local compute-like capabilities include low latency, reliability, reduced backhaul and data localisation, while cloud compute-like properties are flexibility, light device and mobility. As edge computing will likely be more expensive than cloud, only the use cases that require a mixture of those capabilities to run an application create a business case. This situation can be described as the “edge sweet spot”.
What are some examples of use cases that have edge sweet spot requirements?
There are various use cases that would benefit from using edge computing, across a range of industries from agriculture and extractives to manufacturing and healthcare. This article will give a brief overview of three use cases, namely advanced predictive maintenance, remote patient monitoring and video ingest and analytics for security, all of which require a blend of local compute-like and cloud compute-like capabilities. However, the list is by no means limited to these and more use cases can be found in our Edge Computing Use Case Directory.
#1 Advanced predictive maintenance
One of the use cases that could benefit the most from edge computing is advanced predictive maintenance. To accurately assess the condition of an asset, large quantities of data from sensors installed in the machinery need to be processed, which is enabled by edge. This allows firms to move from fixed maintenance schedule to an “as needed” one, which limits costly downtime and increases return on assets (up to 24%).
Some of the capabilities required to enable advanced predictive maintenance solution (e.g. mobility, flexibility and light device) could be supported by running the application in the cloud. However, putting the application at the edge can give businesses a competitive advantage through better data processing and increased speed. Sending data from thousands of sensors to be analysed in the cloud comes with potential latency issues, which can cause delays in response time. As analytics for advanced predictive maintenance need to be done in real time to provide an accurate picture of an asset’s condition, it requires more stringent latency, which can be supported by edge. Additionally, edge computing could be more cost-effective, as it minimises the volume of data that needs to be transferred to the cloud, reducing the transmission and cloud storage costs. As the data is kept on premises, enterprises can also be certain that proper data security is maintained.
#2 Remote patient monitoring
Another use case that would benefit from the sweet spot of edge computing is remote patient monitoring. To reduce the pressure the healthcare sector is facing nowadays, patient wearables, IoT and e-health devices can be used to monitor the person’s health in real-time and notify practitioners if there is cause for concern, for instance, increase in blood glucose levels or dangerously elevated heart rate. This allows doctors to maintain the same quality of care but without taking up hospital resources.
The main issue with implementing this use case is data sovereignty and security concerns. Running the application at the edge can solve this by allowing data to be processed in country, rather than transmitting it to the cloud, which ensures the security of sensitive information. In addition to this, using edge is a more cost-effective option as it reduces backhaul costs of continuously sending data to the cloud. As the data is processed locally, the delays between detecting an anomaly and triggering an alarm are minimised, allowing the healthcare providers to respond to changes in patient’s condition in real time, which guarantees quality of care.
In addition to the local compute-like capabilities, enabling remote patient monitoring requires some cloud compute-like capabilities to ensure the comfort of the patient. The devices must be light and comfortable to wear, so the patient is able to use it constantly without taking off. The device also needs to be mobile to allow the patients to freely move around.
Find out more about digital health in our article: Digital health at the edge: Three use cases for the healthcare industry.
#3 Security: video ingest and analytics
In our Edge computing market sizing forecast, we estimated that video analytics will be a major driver for edge adoption. One of the most mature use cases today is video analytics for security.
The main requirement for video analytics for surveillance is low latency, which cannot be achieved by putting an application on cloud. As such, running an application at the edge would allow the data stream to be analysed near the origin point, hence saving the time of routing the video traffic back and forth and reducing the burden on network connectivity. This would also enable other functions, such as aggregation and filtering of video streams, as well as real-time alarms and actioning. Besides providing low latency and reduced backhaul, edge cloud maintains data security and sovereignty that public cloud cannot, which is essential when storing and managing particularly sensitive data, for example, from facial recognition.
In contrast to local, proprietary infrastructure (e.g. the processing happening on a smart video camera device itself), edge architecture offers the flexibility and scalability of the cloud native solution. This allows simple (and therefore cheaper) cameras to be used because the analytics is run at the edge rather than on the camera. This makes the solution more cost-effective and lowers the barrier for adoption, as any end camera system can be utilised (even an already existing one).
Find out more about video analytics in our report: How video analytics can kickstart the edge opportunity for telcos.
Author: Gabija Cepurnaite is a consultant at STL Partners, specialising in edge computing and MEC APIs.
Read more about edge computing
Edge computing market overview
This 33-page document will provide you with a summary of our insights from our edge computing research and consulting work:
What does IPCEI CIS tell us about the future of edge and cloud in Europe?
We discuss this EU-funded initiative’s pivotal role in advancing interoperability, sustainability, and cybersecurity, as industry giants like Orange, SAP, and Deutsche Telekom collaborate to shape Europe’s tech trajectory.
Accelerating the network: Lessons from Lumen and SK Telecom
STL Partners has conducted extensive research on telcos globally, focusing on their edge computing deployments. STL’s report “Telco network edge computing: Lessons from early movers” delves into the experiences of Lumen, SK Telecom, Telefónica, Verizon, and Vodafone, all of which commercialised edge nodes before 2020. This article highlights key findings and four crucial insights gleaned from these early telco-edge movers.
15 Edge AI Companies: Independent Software Vendors (ISVs) to watch in 2024
STL Partners look at 15 Independent Software Vendors (ISVs) that are using edge computing technology to ensure maximal performance and reliability in their AI models.